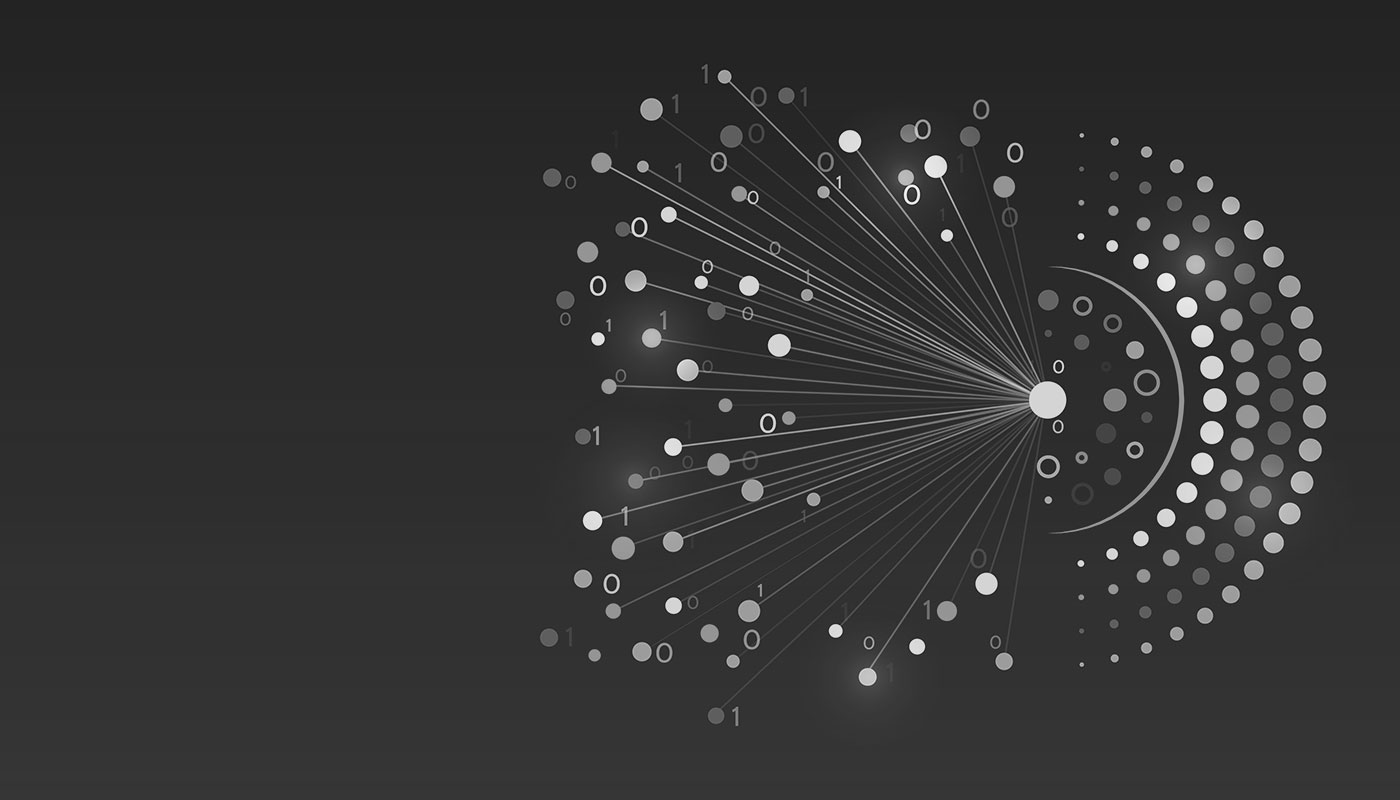
Large data can offer a massive affordable advantage for companies. Scientists, information analysts, marketing professionals, and advertisers rely upon receiving valuable insights from substantial pools of consumer information. When examined correctly, this information can provide valuable insight for organizations that understand how to use it.
The regular procedure of gathering and arranging massive datasets can be taxing, as well as resource-intensive. The privacy issue posed by the collection of consumer data is one of the most challenging of all. To address these growing problems, the use of synthetic datasets is a new approach that is gaining traction.
What are synthetic datasets?
Synthetic datasets consist of information that mimics the statistical properties of real-world data. This simulated information uses pertinent details while covering up the privacy of the individual from whom the data originated. Artificial information offers numerous benefits for performance, scalability, safety and security, and privacy.
Organizations can safeguard as well as improve their existing data with artificial datasets. These synthetic datasets can be used in accordance with data governance best practices.
Advantages of Synthetic Datasets
Scale efficiently
Synthetic datasets are cost-effective and efficient solutions. Data teams can replicate synthetic data that resembles the target population or consumer demographic. Businesses, in particular, can rest easy knowing that if there ever is a massive data breach, none of the data affected will be able to be personally damaging to their customers or employees.
Synthetic data can also be used to populate existing datasets if there is a lack of usable data. Similarly, synthetic data can be sold without leaving the source of the data collection feeling ethically unsure. It can also develop models for AI or other purposes. It also reduces the need for massive, time-consuming data collection efforts.
This approach accelerates the data analysis pipeline. It also enables teams to rapidly prototype and test models to keep ahead of organizational development expectations. Furthermore, it saves precious time. There are fewer constraints imposed by not having enough data or not having real-world or real-time data.
Data Governance Best Practices
While leveraging synthetic datasets offers benefits, it also has some challenges as well. For example, it’s crucial to adhere to data governance best practices to ensure ethical and responsible use of the simulated data. There are a few key considerations that can help enhance cybersecurity levels and avoid a data breach.
Data Privacy and Security
Synthetic data should be generated in a way that preserves privacy and security. The generation process should ideally delete forever any direct or indirect identifiers that could potentially compromise the personal privacy of anyone involved. Organizations must make sure that the generator they use adheres to privacy regulations.
Diverse Data and Coverage
Real-world datasets may suffer from biases or inaccuracies based on human error. They may also suffer from data scarcity or lack of context. Synthetic datasets can address these problems by providing larger chunks of data that fulfill a wider range of realistic scenarios. This results in optimal result accuracy.
Conducting data-centric research in this manner is the key starting point for any developer. It doesn’t matter the specific niche that they choose to work in - data can be even more important in that scenario of limited data.
Transparency and Methods of Documentation
Documentation and transparency surrounding the generation process are crucial aspects of maintaining accountability and facilitating reproducibility. Data teams must document the synthetic data generation methodology in laborious detail.
This recordkeeping will help ensure transparency and protect data analysts in the future if a prediction or pattern proves false. It can also enable others to understand the limitations and potential biases associated with the synthetic data - a huge factor to consider and one that will be more important in the future. It’s important to realize that even accurate and extensive data is not foolproof in predicting the best courses of action or how future consumers will behave.
Quality Control
Synthetic datasets must be evaluated to ensure their statistical properties align with the original data. Data teams should use extensive quality assessment techniques and variations, including statistical analyses and model performance evaluation. This is meant to validate the effectiveness of the synthetic data in truly and accurately predicting real-world patterns so that they can be used in business decisions.
Potential Use Cases
Model Testing and Validation
Synthetic datasets are completely necessary for testing and validating AI or machine learning models in scenarios where real data may be limited or is in the process of being gathered. Real data can also be used alongside synthetic data in order to obfuscate the data set, blending them together into a collection of purely logistical data.
By mixing real data with synthetic data, data scientists can perform extensive testing and “stress-test” their models with diverse scenarios. They can also compare and contrast results this way. This can help assure the robustness and general nature of the models being used. It can also prepare the same models for future real data if needed.
Open Source Synthetic Data
Synthetic data can serve as a privacy-preserving alternative for data sharing. It can be used in open-source projects without worrying about the need to encrypt it. Instead of sharing real datasets which may contain sensitive information, organizations can distribute mock data that is still meaningful. This data mirrors the statistical credentials of the real data, while still protecting individual privacy. This facilitates collaboration and knowledge sharing without compromising privacy or data security - a big win-win for everyone.
Helping with Data-Intensive Research
Synthetic datasets are particularly valuable in domains where data collection is pricy, time-consuming, expensive, or ethically challenging. Many industries work with extremely sensitive data. For instance, in healthcare, data is valuable for research but can put patient privacy at risk. Synthetic data can be generated to mimic patient populations. This can equip medical researchers to conduct in-depth studies without violating HIPAA privacy regulations or compromising their patient confidentiality while building trust.
Generator Capabilities
The methodology behind synthetic data generation depends on the specific requirements of the data being used. It also depends on the characteristics of the way the data is stored, which is why we won’t go too in-depth in terms of the methodology here. However, various techniques can be employed. Generative Adversarial Networks (GANs), Variational AutoEncoders (VAEs), and rule-based generators are all popular techniques. Whatever generator you choose, please consider the following factors:
1. Generator Training
Training is important - generators require sufficient training to accurately replicate the statistical properties of the original data. Ensure that the generator has been trained on a relevant dataset that adequately represents the target population before deploying. The training method is probably the most important factor in creating synthetic data.
2. Data Complexity
Some generators may be better equipped than others for your specific needs. Some may be better suited for certain types of data, such as images, text, tabular data, or audio or video clips. What type of data do you rely on for your research?
The generator's performance and capabilities should be evaluated following the data types and features relevant to your use case. Using a lot of different types of media can make developing a generator a bit more of a challenge, of course.
3. Custom Control
Different generators offer varying degrees of customization. More control is better when dealing with highly sensitive or influential data. Consider whether the generator allows you to implement specific rules. Do you want to simulate different scenarios or generate data with specific characteristics that align with your analysis requirements? Do you want to have built-in rules to avoid bias or inappropriate pattern detection? Ask yourself these important questions before choosing a generation method for your synthetic data.
Conclusion
Synthetic datasets can help us harness new possibilities for efficient, secure, and scalable data analysis for pattern prediction. Synthetic datasets provide a viable alternative to manual data collection and labeling and are the way of the future. Synthetic data also addresses individual privacy concerns. It also enhances the diversity and coverage of datasets. It can go a long way in ensuring underrepresented groups are included in the data.
However, it is crucial to follow data governance best practices. Organizations must ensure generator capabilities align with use case requirements. They must then perform thorough validation of synthetic data to guarantee its quality and suitability.
With the right approach, synthetic datasets can revolutionize the way data teams analyze data to reach actionable conclusions. This can open the doors to deriving new insights from data, a goal for many organizations to harness in the future. This can foster innovation and advance data-driven decision-making processes in the long run, all while saving time and resources and protecting privacy.
Editor’s Note: The opinions expressed in this guest author article are solely those of the contributor, and do not necessarily reflect those of Tripwire
Meet Fortra™ Your Cybersecurity Ally™
Fortra is creating a simpler, stronger, and more straightforward future for cybersecurity by offering a portfolio of integrated and scalable solutions. Learn more about how Fortra’s portfolio of solutions can benefit your business.