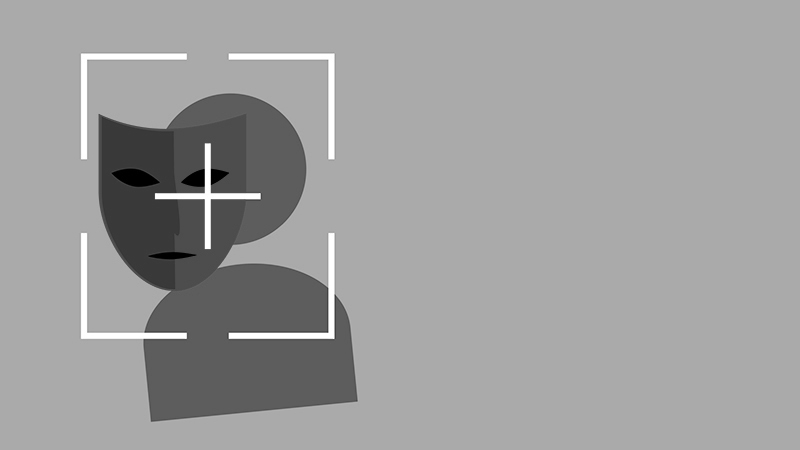
Deepfakes are forged images, audio, and videos that are created using Artificial Intelligence (AI), and Machine Learning technologies. According to the World Economic Forum (WEF), deepfake videos are increasing at an annual rate of 900%, and recent technological advances have made it easier to produce them. VMware states that two out of three defenders report that deepfakes were used as a part of an attack to influence operations, or to launch disinformation campaigns. Other uses include phishing scams, identity theft, and financial fraud.
There are many recent deepfake attacks, such as fraudsters using deepfake technology to create a hologram, which was then used in video calls to pose as a chief communications officer, deceiving other executives to disclose confidential information. In instances of using deepfake audio, threat actors have used real-time voice cloning technologies to fool a Hong Kong bank manager into transferring $35 million to the attacker’s organization. In one particularly egregious scam, the threat actors sent emulated voicemails of a CEO, requesting employees to contribute to charitable, disaster relief causes through fake websites that transferred the money to offshore accounts.
Types of deepfakes:
- Textual deepfakes – A text-generating system that can create writing pieces such as articles, poems, and blogs.
- Deepfake videos – Realistic-looking videos generated by AI and video editing technology. This technology is widely available on applications used in smartphones, which are used to swap a person`s face with another face or filter.
- Deepfake images – This technology is also widely used in social media, and real photographs of people can be edited to have different bodies and faces.
- Deepfake audio – There are software programs that can imitate a person`s voice, including its tone and accent.
- Real-time/live deepfakes – Audio and video clones can be generated in real-time, copying someone`s identity. Threat actors can bypass security measures such as voice-based authentication using this technology.
How deepfakes are created?
A machine learning technology called Generative Adversarial Networks (GANs) is used to generate deepfakes. Two neural networks are run in tandem to produce a realistic output. A network called “Generator” produces forged images as realistically as possible, and the second network, named “Discriminator” compares the forged image with the genuine images to determine which are real and fake. The cycle continues until the discriminator fails to distinguish that the generated image is fake.
Another technology using AI is called “encoders”. They are used in face-swapping/face-replacement technologies. Initially, thousands of face images of two people are run through the encoder to find the similarities between them. Then, another AI algorithm, the decoder, takes the images of the faces and swaps them. With this tool, one person`s facial image can be very convincingly merged onto another person`s body.
How to Spot Deepfakes
Deepfakes can be recognized through unusual or unnatural features or movements. Unnatural eye movement, and lack of blinking are clear signs of deep fakes. Replicating natural eye movement through body language is harder for deepfake tools. Often, deepfakes are detectable through unnatural facial features and expressions. Also, the lighting and the facial features of the image or the video such as the hair and teeth may seem to be mismatched. In the most obvious giveaways are misaligned facial expressions, and sloppy lip to voice synchronizations, unnatural body shapes, and awkward head and body positions. Technical deepfake detection methods include hashtag algorithms, block-chained digital fingerprints, and reverse image search engines.
How to counter against deepfake attacks
Deepfake attacks can be limited through social media rules that are created on their platforms. For example, Twitter and Facebook have enforced policies that ban deepfake technologies. Adobe has also developed verification programs to confirm whether a creation is original. Research lab technologies are using watermarks and blockchain to detect deepfakes. Deepfake detection challenges and programs are also expanding the knowledge about tackling deepfakes. Companies now employ centralized monitoring and reporting tactics with strong detection measures to combat deepfakes.
Conclusion
The amount of deepfake attacks is growing consistently. Their authenticity and genuineness are harder to recognize since their technology is growing rapidly. Using AI and machine learning, deepfakes are the hardest threats to combat. It is crucial to understand the threat landscape of deepfakes, and prepare for stronger defenses in the future.
About the Author:
Dilki Rathnayake is a Cybersecurity student studying for her BSc (Hons) in Cybersecurity and Digital Forensics at Kingston University. She is also skilled in Computer Network Security and Linux System Administration. She has conducted awareness programs and volunteered for communities that advocate best practices for online safety. In the meantime, she enjoys writing blog articles for Bora and exploring more about IT Security.
Editor’s Note: The opinions expressed in this guest author article are solely those of the contributor, and do not necessarily reflect those of Tripwire, Inc.
Meet Fortra™ Your Cybersecurity Ally™
Fortra is creating a simpler, stronger, and more straightforward future for cybersecurity by offering a portfolio of integrated and scalable solutions. Learn more about how Fortra’s portfolio of solutions can benefit your business.