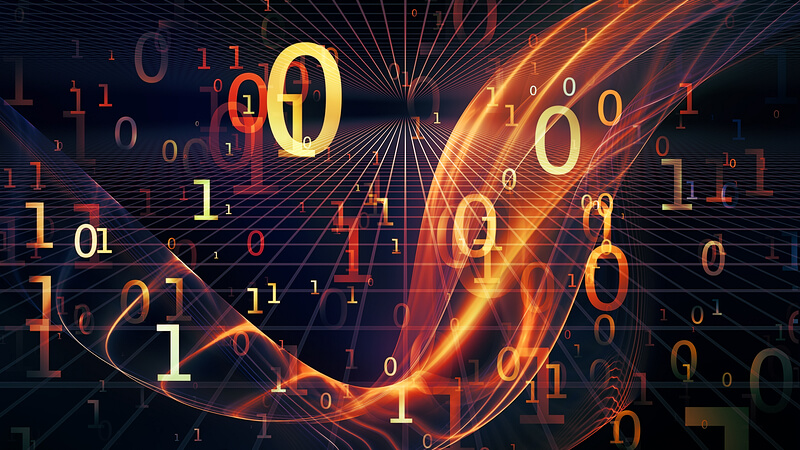
User Behavior Analytics. Network threat detection. The increasing focus on using security data analytics to extract insight and find or predict ‘bad’ has brought with it an influx of marketing promising close-to-magical results. Among the offenders are those machine learning products suggesting data can be thrown at an algorithm and – voila! – insight will appear. The hype around ‘ML’ in a lot of today’s infosec tooling typically obscures the less glamorous, but fundamental, aspect of data science: data collection and preparation (the latter being about 80 percent of a data scientist’s time). The truth is, machine learning and other algorithms need to be applied to appropriate, clean, well-understood data to even return valid results. The fact that there’s misinformation in security marketing is hardly a surprise, but in an infosec context, it can have a pernicious effect. Infosec has so many complex, disparate data sets that use automated analysis to pull them together and make sense of what they mean for different stakeholders (the CISO, SecOps, IT Ops, the Risk Committee). If machine learning products set expectations high and then fall short, lots of data skeptics can be left in their wake – including the people who hold the purse strings to security’s budget. Get it wrong the first time, and you may not get them to buy into a data-driven solution again.
Sense checking security analytics product promises
If you’re buying an analytics/metrics tool that makes big claims about how it can turn your data into gold dust, here are some key pieces of information to obtain before you go ahead:
- What data needs to be ingested by the tool for you to get the promised results?
Some analysis products simply can’t work without data that comes from systems tuned in a certain way. For example, if the platform you’re looking to buy uses web proxy data, what level of logging is required to get the fields it needs compared to what’s actually turned on in your solution right now? Will infosec be able to request increased logging? What about the increased storage required? Others require data from the whole network to give you the visibility you’re looking for. Otherwise, you may be making decisions based only on the alerts you can see, not necessarily on the alerts that matter. The vendor should inform you of how the completeness of information you’ll be making decisions on differs from what’s stated on the marketing material if there’s certain data or data fields you weren’t able to get access to. Also consider the red tape you’ll need to deal with to get access to each different source required. Is the data owned by infosec or by a third-party, such as Infrastructure or an external vendor? Will you be able to get access to it, and in what format – i.e. has the data been modified? This is important because if it has, this can affect the analysis that’s possible. How quickly can you ingest data, and how soon can you ingest it after it’s been created? Can you just pull it from the cloud via an API (e.g. vulnerability data), or will the network team have to move logs across your infrastructure (e.g. active directory event logs)? A lag between data being generated to being ingested may affect your ability to take timely action.
- For how long after installation must this data be collected in order for the tool to achieve the promised level of accuracy/effectiveness, and at what point before this will its output be useable?
Machine learning models need to be trained. For example, for a threat detection tool to find anomalous behaviour in your network, the model must first be supplied with data which covers all the usual network behaviours occurring over time. More data means more power to detect changes that are truly out of the ordinary. The vendor should advise you of any caveats, related to the training of the model, that you should take into account when using the tool to solve a particular problem at a particular point in time.
- What volume of data will the tool output and what else do you need to turn this into ‘results’ that are actionable by your team?
If your new threat detection solution produces 1,500 alerts daily that then need investigation by SecOps, the people to do that need to come from somewhere. Furthermore, has your vendor indicated how many of those alerts are likely to be “true”? Machine learning models will always return “false positives,” so make sure you ask your vendor about the precision (number of true positives/ (number of true positives + number of false positives)) of their algorithm and how much tuning is required to achieve this. If precision is low, your team will be wading through a lot of noise. If it’s high, but the tool requires analysts on the vendor side to do lots of tuning to achieve this, be aware of the dependency you’ll have on their skills. It may not be an issue if you need to ingest seven data sources that are high effort to get access to and move across the network. It may not be an issue if you then need to wait for nine months for an ML model to be trained before you know if your investment delivers a strong ratio of cost to value. But it will be an issue if you don’t know about this when you sign up because these are the factors that will impact the way you approach your investment of time, effort and money – and the way you set internal expectations for what the result of your efforts will be.
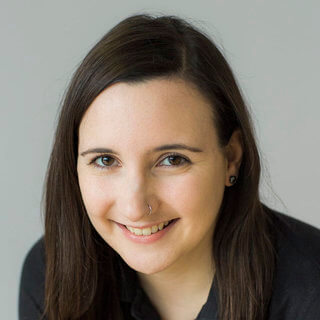
About the Author: Leila Powell is a data scientist working in security. She used to use supercomputers to study the evolution of galaxies as an astrophysicist. Now she tackles more down-to-earth challenges, (yes, the puns get that bad), helping companies use different data sets to understand and address security risk. As part of the team at Panaseer (a London based security start up), she works with security functions in global financial firms, applying data science to help solve strategic and operational challenges. Editor’s Note: The opinions expressed in this guest author article are solely those of the contributor, and do not necessarily reflect those of Tripwire, Inc.